Machine Perception Lab Seeks to Improve Robot Teachers with Intelligent Tutoring Systems
By Tiffany Fox, (858) 246-0353, tfox@ucsd.edu
San Diego, CA, July 30, 2008 -- Just like their human counterparts, robot tutors can rattle off lengthy lists of Spanish vocabulary or teach schoolchildren how to count in increments of 10. But there's one important thing most robot tutors can't do: Recognize when students are getting bored or confused, and modify their lessons to suit.
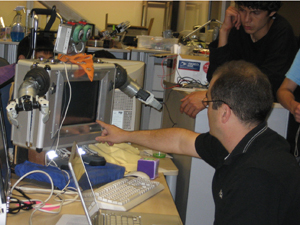 |
Machine Perception Lab Co-Director Javier Movellan operates RUBI, a robot tutor equipped with an Intelligent Tutoring System that teaches pre-school children colors, shapes, and even Finnish vocabulary words. |
|
Researchers in the UC San Diego Machine Perception (MP) Lab are trying to narrow that learning gap by improving what are known as Intelligent Tutoring Systems (ITS). ITS are computer systems that can simulate a human teacher by providing direct, customized instruction or feedback to students without the intervention of human beings. To make the systems more effective, the MP Lab -- which is part of the Institute for Neural Computation and is housed at the UCSD division of the California Institute of Telecommunications and Information Technology (Calit2) -- is studying the feedback that human students provide while learning, be it through facial muscle movement, head positioning or other expressions of interest or confusion.
"Our main goal is to improve robot teachers with these intelligent tutoring systems," says Jacob Whitehill, a Ph.D. student in computer science and engineering at UCSD's Jacobs School of Engineering, and a researcher at the MP Lab. "In recent years, more effort in the ITS community has been spent on making ITS systems more 'affect-aware', or in other words, aware of the emotional state of the student. If the robot teacher is receptive to the students, if they back-up and review when necessary, if they answer questions and ask students questions, then the students feel more involved. And when they're involved, they're far more interested and learn more efficiently."
ITS have been pursued for more than 30 years by researchers in education, psychology and artificial intelligence, and both operational and prototype systems provide instruction in schools and in corporate and military training. Unlike a typical computer-based training simulation, however, ITS assess each learner's actions and develops a customized "learner model" of their knowledge, skills and expertise.
The MP Lab is primarily concerned with ITS for education, since machine teachers equipped with ITS have enormous potential for improving students' academic performance. The lab’s work in ITS is funded by the UCSD-based and Calit2-affiliated Temporal Dynamics of Learning Center (TDLC), which was established in 2006 through a grant from the National Science Foundation. The TDLC seeks to understand the role that timing plays in the functioning of certain regions of the brain when a person is learning new facts, interacting with colleagues and teachers and experimenting with new gadgets.
The lab works in close collaboration with the TDLC, says MP Lab co-director and research scientist Javier Movellan.
“Our work is certainly influencing and being influenced by other TDLC projects,” he says. “For example we are starting a new TDLC project in which we will be adapting ITS technology to test whether it can be used to teach children with autism basic facial expression production and facial expression recognition.”
Despite the endless roles robot teachers can play in the classroom, Whitehill insists that ITS are not meant to replace human instructors. Instead, ITS-equipped robots are primarily designed to assist teachers with simple lessons, giving their flesh-and-blood counterparts the time they need to delve into more complex material.
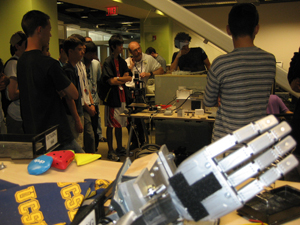 |
High school students get a tour of the Machine Perception Lab, where researchers employ robot teachers to tutor students in basic subjects. |
|
"Robots can assist human teachers with mundane tasks like teaching vocabulary word memorization or other rote exercises, and in areas of the world where there are few teachers, robots could be made available in certain circumstances," Whitehill says. "The more intelligent perception capabilities you endow it with, the more it can approximate a teacher. But I don't think humans have to fear for their jobs just yet."
The benefits of academic tutoring -- whether facilitated by a robot or a human -- have been well-documented. In the mid-1980s, educational psychologist Benjamin Bloom concluded that students who receive one-on-one instruction perform two standard deviations better than students who receive traditional classroom instruction.
Notably, some research suggests that ITS might be more effective than human tutors. Around the same time that Bloom was conducting his study, researchers at Carnegie Mellon University developed an ITS called the LISP Tutor that taught computer-programming skills to college students. In one experiment, students who used LISP scored 43 percent higher on the final exam than a control group that received traditional instruction, and the LISP users required 30 percent less time than the control group to solve complex programming problems.
Another example: In the early 1990s, the U.S. Air Force developed an ITS called Sherlock to train personnel on jet aircraft troubleshooting. After 20 hours of instruction, learners taught by Sherlock performed as well as technicians who had four years of on-the-job experience.
Studies have shown that if a robot tutor is affect-aware and can tailor its lesson to better meet students' needs, students benefit even more from the instruction. With that in mind, the MP Lab has focused much of its research on improving the "instructor model" component of ITS. The instructor model encodes instructional methods that are appropriate for the learner, tracks the learner’s progress and makes inferences about his or her strengths and weaknesses. More and more, those inferences are based on facial expression recognition technology.
The MP Lab's own research into the benefits of ITS and facial expression recognition comes by way of a funny-looking, bandana-wearing robot named RUBI (Robot Using Bayesian Inference), who interacts with children at UCSD's Early Childhood Education Center, teaching them numbers, colors and other basic concepts (even, during one research period, Finnish vocabulary words). RUBI is equipped with a Computer Expression Recognition Toolbox (CERT) that includes "smile detection" technology -- when RUBI sees a child smiling, she giggles and encourages the student to carry on with a lesson. This smile detection technology led to a smile-learning algorithm, which was derived using a dataset of more than 60,000 individuals. That algorithm later turned up in Sony Shutter Smile Technology, which is found in their latest generation of consumer digital camera products.
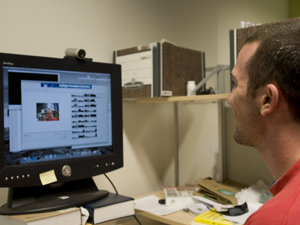 |
Machine Perception Lab researcher Jacob Whitehill demonstrates a facial remote control that he designed as a proof-of-concept ITS application. The device recognizes facial expressions (like Jake's smile, above) and adjusts the speed of pre-recorded video footage to suit. |
|
In the future, other "perceptual primitives" -- simple facial expressions like head shakes that humans perceive naturally -- will be deployed via RUBI to "augment her brain, Whitehill says.
At the MP Lab, teaching robots like RUBI to recognize human facial expressions requires a large database of images, many of which are culled from the Web and then labeled by researchers, who note the head position of the person in the image, for example, or determine if they are smiling or not.
Facial expression recognition software recognizes fine-grain muscle movements of the face based on a framework called a Facial Action Coding System, created in 1979 by UC San Francisco psychologists Paul Ekman and Wallace Friesen. The system delineates 46 different muscle groups of face, including 'inner eyebrow raiser,' 'nose wrinkler,' or 'dimpler,' all for specific muscle groups.
Explains Whitehill: “Expressions that make a larger change in appearance to the face are easier for a robot to recognize. For example, a smile makes a larger change in the face -- it often exposes the teeth, and it can affect the wrinkles around the eyes. In contrast, there's an expression called 'eye widener' where the white of your eye becomes more exposed. Now, that's quite subtle. The less of your face that changes, the harder it's going to be for a robot to recognize."
"Some other kinds of facial expression data are not readily obtainable from the Web," he adds. "And those expressions are very hard to produce in isolation, because it's difficult to tell people to do facial expressions that don't arise commonly. We have collaborated with researchers in psychology to elicit these kinds of facial muscle movements, and they go to considerable effort to design experiments with human subjects so that something like 'pulling back of the mouth corners' might arise naturally.
"What we do not do is tell a robot this: 'A smile occurs when the teeth become more exposed and the mouth corners become drawn out.' Instead what we do -- and this is a data-driven, machine learning approach -- we give many examples of the expression we're looking to recognize, as well as examples that do not contain that particular expression. We then create algorithms that decide the statistical properties of the faces and distinguish between the expression we're looking for and the absence thereof."
Whitehill says it's important within the context of this research to differentiate between "facial expression" and "emotion." Recognizing an expression and subsequently interpreting an emotion represent two separate steps in programming ITS: First, the robot must understand the visual cues and second, it must modify the lesson based on the student's perceived emotions.
"To infer the emotion on someone's face requires knowledge of the context in which the person is interacting," he says. "Facial expression is more of a physiological thing; it's what the muscles are doing. So the first stage is the raw expression, and the second stage is interpreting that expression and determining, in some automated way, what emotion it represents. If a student nods, does that mean they understood the lesson? Are they just bobbing their head up and down? Are they falling asleep?"
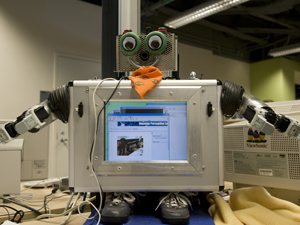 |
RUBI is one of the projects co-sponsored by the UCSD Temporal Dynamics of Learning Center. |
|
As a means of investigating this process, Whitehill developed a proof-of-concept application for a “facial remote control” that recognizes certain expressions students might exhibit during a lecture (smiling to show interest, for example, or frowning to show confusion) and then modifies the speed of a pre-recorded lecture according to those perceived expressions. When a student smiles, for example, the videotaped lecture fast-forwards. When he furrows his brow, it slows down.
Whitehill says he came up with the idea for the device after watching a particularly boring lecture.
"It led me to investigate two questions: Can facial expression predict my preferred viewing speed of a lecture, and also can it predict how easy or difficult I find the lecture to be?"
Adds Whitehill: "When I'm giving a lecture either one-on-one or to a group, I have some intuitive feeling of whether my students are following me based on how they're looking at me. Sometimes they squint and I can tell they're really struggling, and sometimes they're nodding or smiling at me and intrigued for more, and I feel I can keep feeding them information. I think facial expression is a useful feedback signal, certainly between human students and their teachers. But also, it can be harnessed by a machine teacher to make more effective intelligent tutoring systems."
But no matter how advanced their ITS "brains" become, Whitehill says there are some things machine teachers might never be capable of doing.
"Putting your heart into teaching, wanting to help your students and make them feel good about learning -- that is not easily replicable by any kind of hardware," he says. "But it makes a big difference as far as motivating students and giving them a passion to learn."
Media Contacts
Tiffany Fox, (858) 246-0353, tfox@ucsd.edu
Related Links
"Robot Teachers," Voice of San Diego
Machine Perception Laboratory